Goal: Consumption Forecasting
Data
27.300 instances for building (7 buildings)
Sample time: 1 hour.
Consumption data.
Weather data (temperature, solar radiation, relative humidity, etc.)
Occupancy data.
Calendar data (hour, day of the week, month, etc.)
Indoor ambient data (indoor temperature, indoor relative humidity, indoor light level).
Method
-Pre-processing: missing values, feature selection, instance selection, outliers filter, normalization, etc.
-Modelling: multiple linear regression (MLR), neural networks (NN) and support vector regression (SVR).
-Validation: correlation coefficient (CC), mean absolute percentage error (MAPE), etc.
Results
Best predictions around 5% of MAPE.
The electric grid is evolving. Smart grids and demand response systems will increase the performance of the grid in terms of cost efficiency, resilience and safety. Accurate load forecasting is an important issue in the daily operation and control of a power system. A suitable short term load forecasting will enable a utility provider to plan the resources and also to take control measures to balance the supply and demand of electricity. The aim of this software is to forecast the electric load in non-residential buildings. Based on the results, there is the possibility to analyse what kind of data, as weather, indoor ambient, calendar and building occupancy, is the most relevant in building load forecasting. In addition, using the software, the effectiveness of the implemented improvements can be studied. The software can perform predictions with three different models such as MLR, MLP and SVR. The results, from a real case study in the University of Girona (Catalonia), show that the proposed forecast software has high accuracy and low computational cost.
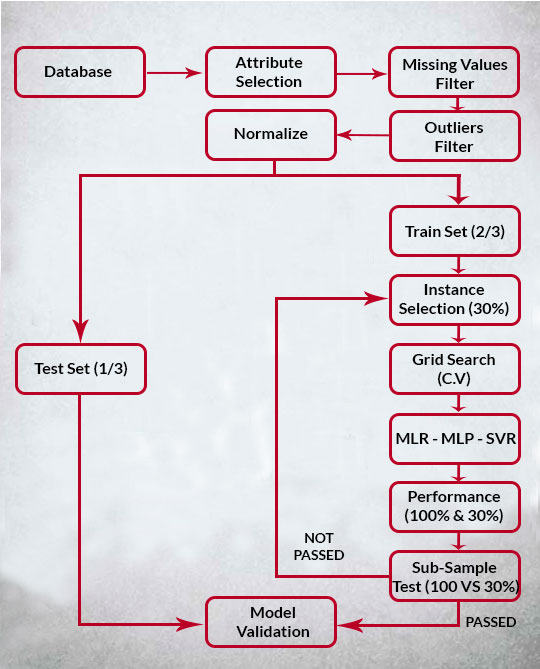
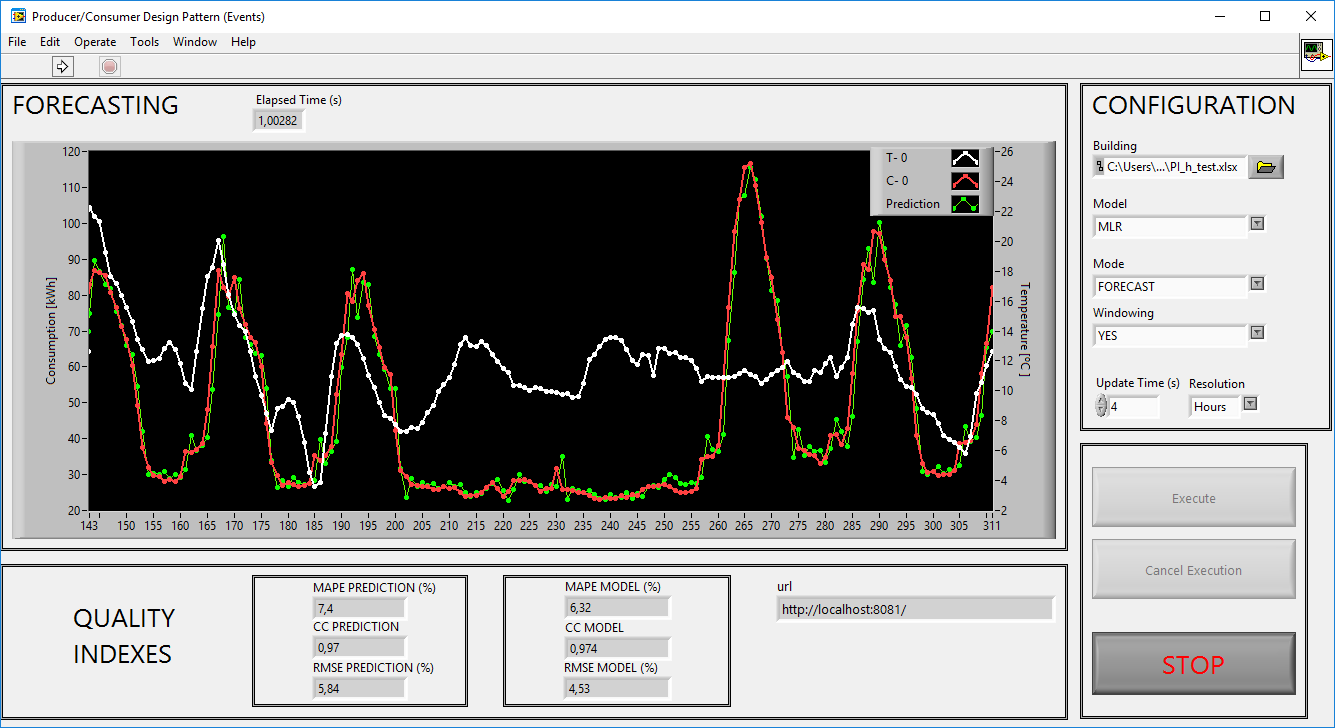