Goal: Personalized diabetes prediction
Data
1074 cases (healthy, diabetes)
Clinical data: IMC, sex, age.
Polymorphisms (112): insulin-like growth factor binding protein, CD36 antigen, adiponectin receptor 2, etc.
Method
Dimensionality reduction with PCA and personalized prediction with CBR.
Results
5 principal components, PCA-CBR success: 89%
Medical databases usually consist of a great number of variables. It is therefore tedious for the diagnostic system to cope with all these attributes. Hence, it is important to find methods to reduce the total amount of attributes without the loss of performance. One of the well-known techniques that has been widely applied to reduce the dimensionality is the principal component analysis (PCA). PCA techniques are designed to deal with numerical attributes, but our medical dataset contains many categorical data and alternative methods such as RS-PCA are required. Thus, we use RS-PCA (regular simplex PCA) suitable for discrete data. We hybridize it with a CBR system to support medical diagnosis. Results are quite promising since they allow diagnosis with less computation effort and memory storage.
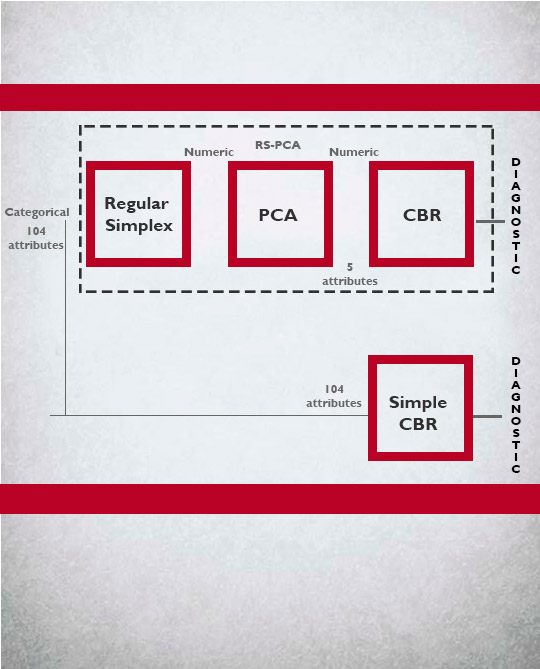