Goal: Breast cancer prognosis
Data
347 families (GED files) with gene mutation information.
554 clinical data of individuals of families (csv files).
Method
Automatically generate inheritance indicators, use indicators with clinical data to predict cancer risk, case-based reasoning for individual-personal prediction.
Results
8 indicators, 11 inheritance rules, 91 % sucess with mutation inheritance. exitCBR tool.
Breast cancer has been our true collaboration boot in the world of healthcare, challenging us with problems that require tools to guarantee experimental repetition, due to the criticality of the impact of healthcare results. To that end, we develop the eXiTCBR tool based on the case-based methodology, since this is the AI methodology that supports evidence-based medicine. Moreover, it allows the hybridization of other machine learning and reasoning techniques. Nowadays, the tool has growth and there are several versions available. Regarding breast cancer, we developed as part of the tool a new reuse method for case-based reasoning, a genetic algorithm to learn the relevance of clinical variables. A plug-in to manage data files inherited from GED has also been developed, so that family inheritance indexes can be managed at the clinical level. Subgroup discovery techniques have also been applied.
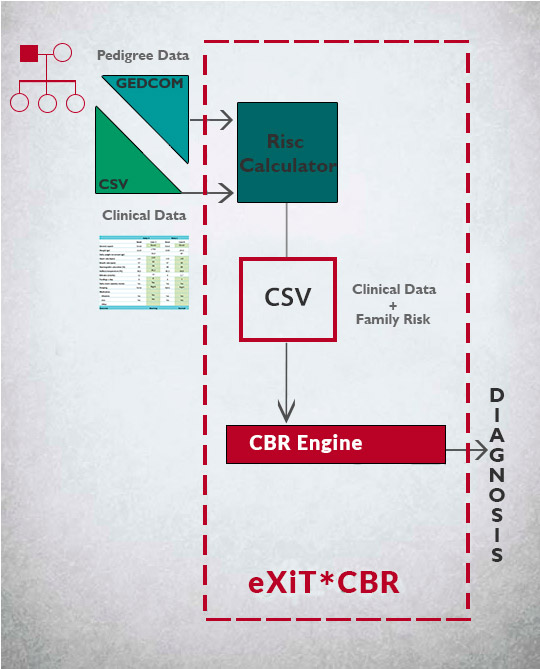